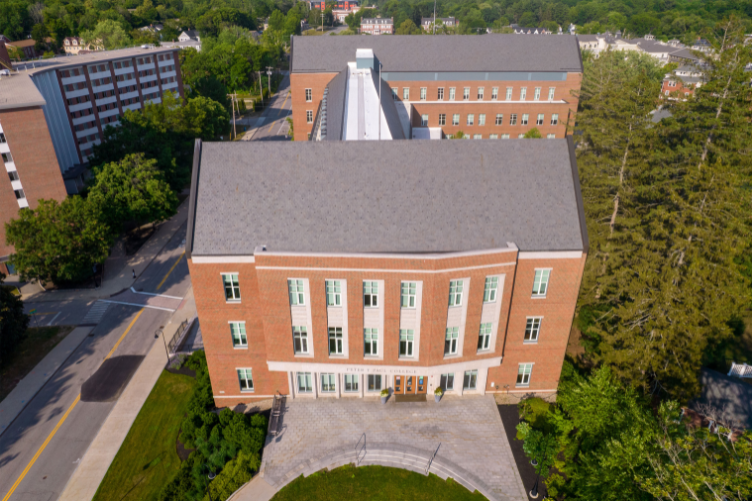
Could machine learning models help the public better judge the quality of the health news they consume? Recent research by two University of New Hampshire professors suggests they can. ?
Ermira Zifla and Burcu Eke Rubini, assistant professors of decision sciences at the UNH Peter T. Paul College of Business and Economics, recently trained machine learning models to evaluate the quality of health news stories about new medical treatments. ?
Their work, published in Decision Support Systems, found that the machine learning models outperformed laypeople evaluations in assessing the quality of these health stories. ? ??
The research tackles the complex challenge of determining the reliability of news that can be more nuanced – instances where the whole story isn’t being told but doesn’t fall into the category of fake news.
This challenge can be more pronounced with the quick and wide dissemination of news stories and press releases about new medical treatments because such stories can feature inflated claims and suppression of associated risks. At the same time, most ordinary people don’t have the medical expertise to understand some of these complexities. ? ?
“The way most people?think about fake news is something that's completely fabricated, but, especially in healthcare, it doesn't need to be fake. It could be that maybe they're not mentioning something,” Zifla says. “In the study, we’re not making claims about the intent of the news organizations that put these out. But if things are left out, there should be a way to look at that.” ??
In their research, Zifla and Eke Rubini utilized a data set from Health News Review that included news stories and press releases on new healthcare treatments published in various outlets from 2013 to 2018.? ? ?
These articles were already evaluated by a panel of healthcare experts – medical doctors, healthcare journalists and clinical professors – based on 10 different evaluation criteria the experts had developed.?The criteria included cost and benefits of the treatment or test, any possible harm, the quality of arguments, the novelty and availability of the procedure and the independence of the sources.
The researchers then developed an algorithm based on the same expert criteria, and trained the machine models to classify each aspect of the news story, matching that criteria as "satisfactory" or "not satisfactory." The expert evaluations were the benchmark against which the machine-learning models were trained and tested. ? ?
“We had this great data set that had news that was evaluated on different criteria, and that is rare because it's costly and requires a lot of time and expertise to do,” Zifla says. “We figured we could leverage that data set and machine learning to automate the process.” ? ?
Their approach, using multi-criteria expert evaluations, contrasts with previous studies that typically rely on a binary true-false framework for detecting fake news, Eke Rubini added. ?
The model's performance was compared against layperson evaluations obtained through a survey where participants rated articles as "Satisfactory" or "Not Satisfactory" based on the same criteria. The survey revealed an "optimism bias," with most of the 254 participants rating articles as satisfactory, markedly different from the model's more critical assessments.?
“We can speculate as to why, but we don't test that in the paper,” Zifla says. “It could be a general tendency to trust the news or medical information.” ??
As the public continues to consume health news rapidly through social media, Eke Rubini and Zifla believe it would benefit social media companies to use multi-criteria machine learning models like the one they developed to create digital nudges to help consumers assess these stories. ??
“Every time a news article comes in about health news, it would run through the algorithms and then give results based on whether it satisfies or doesn't satisfy the different criteria, and that could be incorporated into websites automatically,” Eke Rubini says. ??
Future research could focus on developing new models with different criteria or explore the public’s receptiveness to machine learning evaluations if implemented by social media platforms. ?
“This is a very difficult challenge. We hope to start a conversation about evaluating news based on multiple criteria. I can't emphasize enough that we should move away from the binary thinking fake news or not fake news,” Zifla says. “These models can be adapted with better criteria and better features. This could always be improved.”?
-
Written By:
Aaron Sanborn | Peter T. Paul College of Business and Economics | aaron.sanborn@unh.edu